Why is it important to characterize & quantify Aβ plaques?
Amyloid dysregulation is widely recognized as playing a central role in the progression of Alzheimer's disease (AD) (Selkoe, 2016). Hallmark neuropathologic features commonly seen in AD include extracellular amyloid-β (Aβ) plaques, astrogliosis, and microglial activation (Cohen, 2015). Amyloid plaques present a wide range of morphologies and neuroanatomical distributions. Both immunohistochemistry (IHC) and immunofluorescence (IF) can be employed to obtain detailed information about the localization, distribution, and morphology of Aβ plaques within specific brain regions, providing a level of detail not achievable with other quantification methods (e.g. brain imaging, fluid biomarkers).
Furthermore, clinical data suggests that studying only the global amyloid load in the brain may not give the full picture of the state of the pathology as multiple post-mortem studies have shown patients with abundant amyloid deposits at death without significant cognitive impairment (Katzman, 1988; Hulette, 1998; Aizenstein, 2008). Increasingly, scientists have attempted to link the presence of specific beta-amyloid plaque subtypes with clinical progression, and, for instance, have found that amyloid plaques lacking fibrillar structures (also called “diffuse plaques”) are often found in cases without cognitive impairment, in contrast to more compact fibrillar plaques that are more likely associated with cognitive impairment. The presence of swollen or dystrophic neurites in close proximity to the plaque has also been shown to be strongly correlated with cognitive impairment and disease severity (Dickson, 2001; Ly, 2011). These findings highlight the need for accurate characterization of the various amyloid plaque subtypes, which could help further our understanding of disease heterogeneity.
What are the various subtypes of amyloid-β plaques?
Amyloid-beta plaques originate from the cleavage of the amyloid precursor protein (APP) by the β- and γ-secretase enzymes. This process generates Aβ peptides, which can vary in length, with Aβ40 and Aβ42 being the most significant forms in AD. Aβ accumulation in the brain follows a specific spatiotemporal pattern, initially appearing in the neocortex and eventually spreading to subcortical regions, including the hippocampus (Braak, 1991; Braak, 2006). The Aβ peptide initially exists in a monomeric state, but can self-aggregate into various forms, such as short fibrillar oligomers, globular non-fibrillar oligomers, and amyloid fibrils that form aggregates known as plaques.
Spatiotemporal pattern of β-amyloid pathology progression (adapted from Braak, 1991).
Aβ plaques are classified, based on their morphology and fibril content, into diffuse and dense-core types using dyes that target the β-pleated sheet structure, such as Congo Red or Thioflavin-S (Serrano-Pozo, 2011). Thioflavin-S-negative diffuse plaques exhibit loose shapes and sizes, and lack a well-defined fibrillar structure. These diffuse plaques are commonly seen in the brains of cognitively-intact, elderly people. Thioflavin-S-positive dense-core plaques, on the other hand, have a compact core comprised of a cluster of extracellular filaments intermingled with surrounding neuronal, astrocytic, and microglial processes (Serrano-Pozo, 2011). These neuritic plaques (NPs) are associated with increased synaptic loss, neuron loss, astrogliosis, and microgliosis (Dickson, 2001; Ly, 2011; Tsering, 2023). NPs are commonly found in the later stages of AD, and have a higher correlation with cognitive decline than diffuse plaques (Haroutunian, 1998). This finding underscores the need to distinguish between diffuse and neuritic plaques when attempting to link β-amyloid burden and disease progression.
Aβ aggregates can also deposit in vessel walls, often referred to as vascular Aβ or cerebral amyloid angiopathy (CAA). These deposits are usually formed from Aβ40 peptides, which are more soluble than the Aβ42 peptides that are commonly found in typical parenchymal plaques. Studies have shown that CAA can be a contributing factor to cognitive decline seen in AD (Greenberg, 2004; Arvanitakis, 2011).
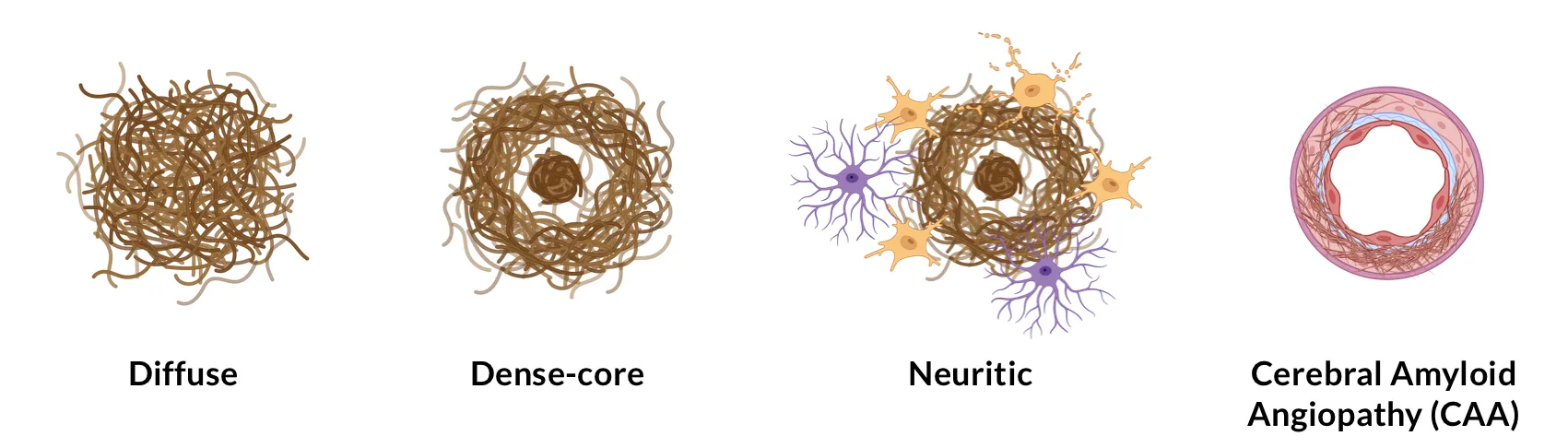
Illustration of various types of amyloid-β plaques.
Other rarer subtypes of Aβ plaques include dense-core coarse-grained plaques, which are associated with the early stages of AD (Boon, 2020), and diffuse cotton wool plaques, often found in patients with specific genetic mutations, such as those in the PSEN1 gene (Crook, 1998).
How are amyloid plaques characterized in IHC and multiplex IF images?
The assessment of plaque burden in immunohistochemistry (IHC) and immunofluorescence (IF) data is conventionally preformed manually using semi-quantitative criteria established by the Consortium to Establish a Registry for Alzheimer’s Disease (CERAD) (Mirra, 1991). These criteria focus on assessing the highest density of neocortical neuritic plaques, and they do not consider the presence of diffuse plaques in the scoring calculation.
Significant efforts have been made to develop quantitative approaches to assess Aβ plaque load that account for anatomical location, plaque density, and plaque subtype. Common methods employed to quantify these features manually include unbiased stereological counts of plaques (Busch, 1997; Ohm, 1997), and grading of plaque densities using a numerical rating scale (Arnold, 1991). These manual assessments can be tedious, prone to interrater variability, and hard to scale for larger studies. Automated methods that rely on classical computer vision algorithms (e.g. thresholding, morphological operations, etc.) have been investigated to reduce the burden on pathologists and increase the reliability of the quantification (Byrne, 2009; Neltner, 2012; Samaroo, 2012; Kapasi, 2023). However, a vast majority of these classical methods still require human-defined inputs, making them susceptible to batch variations and staining variability observed in larger datasets.
Our group has performed quantification of amyloid plaques in a mouse model with early-onset and progressive AD-like accumulation of β-amyloid deposits. This transgenic mouse line (ARTE10) is characterized by overexpression of mutated APP and PS1 (Willuweit, 2009). Aβ plaque load was assessed from IF images of these mice at different timepoints using a combination of blurring, local hysteresis thresholding, and morphological operations. This approach was fully-automated and did not require any human-defined inputs to quantify Aβ load in tissue sections. See our Presentation – Amyloid-β & Inflammatory Microenvironment in Alzheimer’s Mice.
Microscopy Images
The Interactive Image Viewer below allows you to explore the entire multiplex immunofluorescence tissue section.
You can pan around the image using the left mouse button. You can zoom in & out using the mouse/trackpad (up/down) or the + and - buttons in the upper left corner. You can toggle (on/off), change color, and adjust image settings for the channels and segmentations in the Control Panel in the upper right corner.
In this visualization, an APP/PS1 (ARTE10) mouse brain is shown with the "Aβ Plaques" Segmentation Masks (yellow), which can be checked/unchecked to hide/show the underlying "Amyloid-β" staining (red).
Multiplex immunofluorescence stained brain tissue section that demonstrates Aβ plaques (along with segmentation), activated microglia, and reactive astrocytes in a 12 month-old APP/PS1 (ARTE10) transgenic mouse. Note that the sensitivity of the Aβ plaque segmentation can be modified during image processing via various parameter settings and morphological operations.
More recently, research groups have reported using deep-learning approaches for automated classification of AD plaques into plaque subtypes, leveraging the expert-level performance of these models to recognize intricate patterns in images. For example, Tang and colleagues (Tang, 2019) developed a proof-of-concept deep learning pipeline that analyzes whole-slide images, and classifies Aβ pathologies between diffuse plaques, dense-core plaques, and CAA. The same group later expanded on those results by showing that these machine learning models were robust to cohort variations across multi-center studies (Vizcarra, 2020; Wong, 2022). Other groups have reported using similar approaches to differentiate tauopathies (Signaevsky, 2019; Koga, 2022; Wong, 2022). Overall, extensive efforts have been made to train these deep-learning models on a wide range of input parameters (brain region, staining methodology, pathological features) to make them adaptable and generalizable.
Our team would be happy to answer any questions about amyloid-β plaque analysis or provide specific information about the Alzheimer’s disease models that we use for therapeutic efficacy studies.
Discover more about our Alzheimer's Disease Models
Related Content
Up-to-date information on Alzheimer's Disease and best practices related to the evaluation of therapeutic agents in AD animal models.
Astrocyte Morphology in Alzheimer’s Disease
An overview of astrocyte morphological analysis and the applications to neurodegenerative disease research and drug discovery & development.
Amyloid-β & Inflammatory Microenvironment in Alzheimer's Mice
We have analyzed the complex spatial relationships between β-amyloid plaques, activated & resting microglia, and astrocytes in an APP/PS1 transgenic model.
Microglia Morphology in ALS, Alzheimer’s Disease & Parkinson’s Disease
An overview of microglial morphological analysis and the applications to neurodegenerative disease research and drug discovery & development.